What’s real: observation, speculation & counterfactual
This week we kept it light and breezy, with essays about how fiction can help shape reality, how small changes in initial conditions can create wildly different realities, and how what we see may not be real at all. If it’s all a little dizzying for you, stick around for the laser show.
— Alexis & Matt
1: Design for the unreal world
Kicking off this week is an essay from Anthony Dunne and Fiona Raby about the nature of reality and the role of designers in shaping it. For those unfamiliar with their work, Dunne and Raby are renowned speculative and critical designers, using design as a medium to provoke debate about the social and ethical implications of technology. In this essay, they discuss the ways that an embodied fiction can have real impact, and how the delineation we draw between the real and the fake is not as binary as we would imagine. Most importantly, they highlight design’s role in creating imagined possibilities that not only point to alternate realities, but make explicit the ways in which our current reality is intentionally constructed.
“If reality is not given but made, then it can be unmade, and remade. This is not simply about the re-imagining of everyday life—it is about using unreality to question the authority of a specific reality in order to foreground its assumptions and ideology.”
The Designed Realities Studio is a research and teaching platform that combines design with social thought in order to develop alternative narratives to technological futures.
www.designedrealities.org • Share
2: China and alternate internets
Speaking of parallel realities, this New York Times piece on the Chinese internet is a worthwhile read for anyone curious about other ways the internet might have evolved. Specifically, the article dives into the world of WeChat miniprograms and the different online reality they have engendered in China. If you’re not familiar, miniprograms are a lightweight way for any business to easily spin up an app within the walls of WeChat that can leverage many of the platform’s affordances, including built in e-commerce systems.
As a result, the vast majority of digital experiences in China happen inside of WeChat, including not only businesses, but public health care, education, and transportation services as well. On the one hand, it’s a look into an internet in a society whose government has no ethical qualms about a massive monopoly with top-down control. But in other ways, it’s a look into what the internet could have been like elsewhere if payment and commerce capabilities had been built-in and prioritized from the beginning, enabling transactional businesses to grow online earlier and deprioritizing advertising-driven models as the foundation of internet.
“[WeChat miniprograms’] success in China provides a fascinating look into an alternative vision of the mobile internet, one that is integrated across multiple dimensions and that is in essence a single large market. What sorts of innovations does that engender? What sorts of tensions does that create? Is it a better architecture than our Western one, in which each business has its own mobile app, existing in isolation, downloaded but idle for large chunks of the day?”
China’s Internet Is Flowering. And It Might Be Our Future.
What most Westerners don’t know about China’s highly integrated approach to mobile apps: It’s amazing.
3: To fix gender bias, start considering gender?
A now-famous tweet from @DHH (that’s David Heinemeier Hansson, creator of Ruby on Rails and Founder of Basecamp) illuminated the bias in Goldman Sachs’s credit policies for the Apple Card that led him to receive a limit twenty times higher than his wife. While the exact mechanics behind the decision are clouded in its algorithm, it does appear that women get lower credit scores and lower limits than they would otherwise deserve.
Assuming that’s the case, how would we resolve this fairly? It turns out one approach may be via a method that is illegal: considering gender as a signal in the decision-making process. An ongoing study by the UN Foundation and the World Bank found that keeping separate, gender-based data models for credit worthiness tends to mean higher limits and scores for women. Researchers believe this to be because women are, generally speaking, more likely to pay off their loans and credit than men with the same financial resources, so separating women into their own decision models will not penalize them for the behavior of men. However, this still doesn’t explain the underlying disparity in the model that Apple has been using for their credit card, and neither Goldman Sachs nor Apple has been forthcoming with more information on that front.
As we talked about last week, simply splitting applicants into two groups may penalize trans* people and non-binary applicants, and could cause other discriminatory practices. Until then, to properly overcome the inherent biases against women, POC, and other marginalized groups in our historical record, it will be necessary to consider these factors and not pretend that the data itself is blind and unbiased. We can only correct our models and the underlying data by taking this prior discrimination into account.
There’s an easy way to make lending fairer for women. Trouble is, it’s illegal.
Goldman Sachs defended itself in the Apple Card scandal by saying it did not consider gender when calculating creditworthiness. If it did, that could actually mitigate the problem.
www.technologyreview.com • Share
4: She looks familiar, but I can't place her
Facebook AI Research has announced a filter that can alter the facial features of people depicted in videos in order to fool facial recognition systems. This “De-Identification” technique had previously been available for still pictures, but this new technique applies to videos as well and can be applied without retraining the models for a particular video clip. It works similarly to the facial replacement “deep fake” technology that can make a recognizable person say or do something they’ve never done in real life.
This tool has all kinds of altruistic applications, from masking protesters in news footage to hiding the identity of vulnerable witnesses in recorded testimony. More broadly it could allow researchers to use recordings of human movement to train other AI without risking the privacy of the participants. (Currently, large archives of photos and videos are often used to train AI for various reasons, but the facial features of the people in these assets are recognizable.)
As privacy becomes more tenuous with broader facial recognition use (Facebook itself recently made facial recognition the default on its platform for uploaded images and videos) techniques such as these will be a necessary protection for an individual’s identity. It may also spur an escalating arms race between those who want to remain anonymous and those who want to identify them.
Facebook alters video to make people invisible to facial recognition
Facebook AI Research says it’s created the first machine learning system that can stop a facial recognition network from identifying people in videos.
5: The means of (ML) production
This piece from The New York Times describes the increasing gulf between academic research and corporate-owned innovation in machine learning. In short, where academic institutions were once at the forefront of computational innovation as a result of their ability to unite forces from corporations and governments, these projects pale in comparison to the massive capabilities that companies like Facebook and Google have amassed. Given that most ML research today is based on computationally-heavy “deep learning” techniques, those with the most computers will have a huge advantage.
One aspect not discussed as much in this story is the shift toward “deep learning” models that happened in the late 2000s and early 2010s. Prior to this, more heuristic and rule-based models were dominant in AI research, since they required far less computational power. Little progress had been made in making these models work in generalized situations, however, and when GPUs and cloud computing made access to computational power relatively trivial, the more computation-heavy techniques were revisited.
A potential solution to this problem is to continue to find more efficient solutions to common problems, and to continue with pure research into improved learning models that require far less computational power. Aside from the concentration of power in a few corporations, the current models require enormous electrical resources. One study found that training a single AI model could require the equivalent of a car’s lifetime carbon output, so it’s in all our interests to find more efficient ways of teaching our machines to think.
At Tech’s Leading Edge, Worry About a Concentration of Power
A.I. research is becoming increasingly expensive, leaving few people with easy access to the computing firepower necessary to develop the technology.
6: "Neither patients nor doctors have been notified"
Last week, The Wall Street Journal reported on a secret Google project called Project Nightingale, in which it is harvesting tens of millions of personal medical records as part of a machine learning project that aims to build a search and recommendation tool for medical professionals. Most notably, none of the affected patients or doctors were notified of the project. Google claims that they are compliant with HIPAA privacy laws, seemingly because they are claiming to only be a “business associate” that is helping Ascension (their health-care provider partner) provide health services. However, the Department of Health and Human Services is investigating the project to determine whether they are acting legally. There is a strong potential argument to be made that Google’s role is much more like that of a health care provider itself, which would subject it to much stricter regulations.
This is one of many initiatives where tech companies are making forays into other areas beyond the typical boundaries of internet technology companies. These efforts raise questions about what role those companies are performing and how they should be regulated. When Google starts mining your health data, are they a health care provider? When Facebook runs a cryptocurrency or Google offers you a checking account, are they banks? If Alexa starts to offer emotional support based on your tone of voice, should Amazon need to be a licensed therapist?
Google, Project Nightingale, and All Your Health Data
Google is an emerging health-care juggernaut, and privacy laws weren’t written to keep up.
One laser thing
Now that we’ve pointed out all the dystopian ways large corporations often control the application of new technologies, here’s a little palate cleanser. In Chilean protests last week, we saw protesters using lasers en masse to neutralize riot police, in one case even taking down a police drone. So you know, sometimes the people rise up and take tech into their own hands. And sometimes that tech is LASERS.
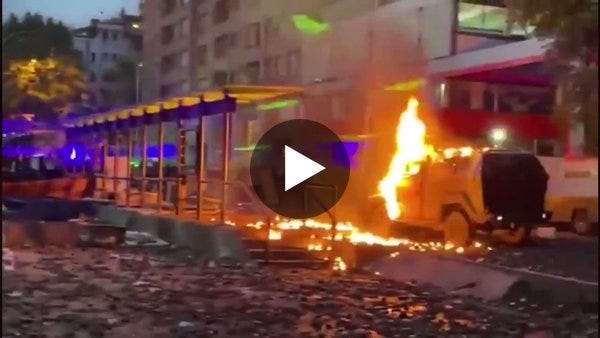
Protesters in Chile employing Lasers en masse to disorient, neutralize Riot Police https://t.co/MsBJLCSZuD
Six Signals: Emerging futures, 6 links at a time.
If you don't want these updates anymore, please unsubscribe here.
If you were forwarded this newsletter and you like it, you can subscribe here.
Powered by Revue